Switch content of the page by the Role togglethe content would be changed according to the role
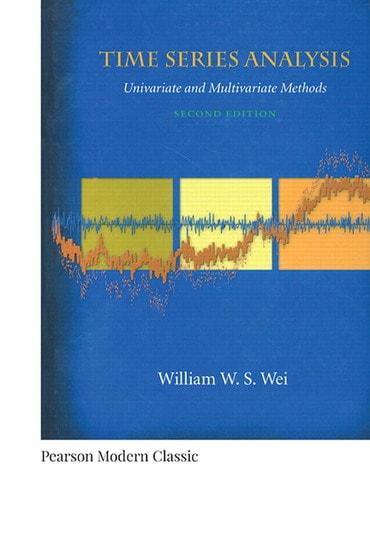
Time Series Analysis: Univariate and Multivariate Methods (Classic Version), 2nd edition
Published by Pearson (May 26, 2023) © 2023
- William W.S. Wei
Pearson+ subscription
per month
-month term,ISBN-13: 9780137981465
Time Series Analysis: Univariate and Multivariate Methods
Published 2023
Paperback
$95.99
Price Reduced From: $119.99
ISBN-13: 9780134995366
Time Series Analysis: Univariate and Multivariate Methods (Classic Version)
Published 2018
Need help? Get in touch